General Information about GPCRs:-G-protein-coupled receptors are a pharmacologically important protein family with approximately 450 genes identified to date. Pathways involving these receptors are the targets of hundreds of drugs, including antihistamines, neuroleptics, antidepressants, and antihypertensives.The GPCRs are consist of seven transmembrane domains that are connected through loops.The N terminal of these protein are located extracellularly and C terminal is extended into the cytoplasmic space. Due to this topology they are able to transduce the external signal into the cell. This transduction of signal took place with the help of G-protein.At present the sequence of more than thousand of GPCRs is available. In recent time ,few more sequence will become available with the completion of genome sequencing projects such as human genome.In contrast the three dimensional structure for these protein is largely unsolved.The structure for bovine rhodopsin is only available at 2.8 Angstrome.The currently available GPCRs are classified to major five classes , which are further classified to subfamilies.The major classes of GPCRs is shown in figure below.
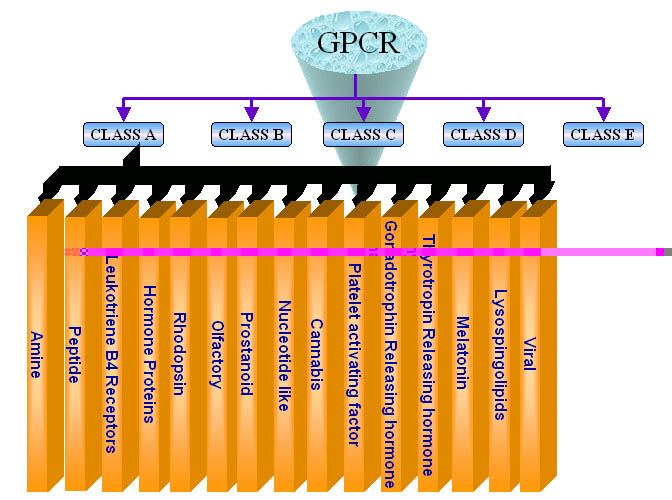
In this era of genomics, the genomic data is produced at exponential rate. The functional and structural annotation of the proteins is an important task in proteomics. their is strong need for efficient and reliable methods for the annotation of proteins. In the past, the similarity search base tools are mostly used to annotate the proteins. These tools fails when there is no significant similarity between the query and proteins of database.To overcome the limitations of above methods, many statistics based methods were developed. In the past only single methods was developed for predicting the subfamilies of GPCRs. The methods is not functional at present.This motivated us to develop a method for recognizing and classifying the GPCRs from the protein data.The major problem in designing a machine learning technique based methods is to obtain the vectors of fixed length. Here, we have developed a SVM based method using the dipeptide composition of proteins.The dipeptide composition is better in comparsion to only amino acid composition based method because dipeptide took in account both the amino acid composition as well as local order of amino acids. we have designed a method using the three step statergy for recognizing and classifying as shown below.

The method can identify the GPCRs with nearly 100% accuracy. The method can predict the classes and sub-families of GPCRs with more than 80% accuracy.In summary, the methods can assist in automated annotation of protein by recognizing the GPCRs from the protein sequences.
Supplementary information
Table_sup1:-The performance of composition based module developed using the dataset of 2872 protein obtained from EVA server.The sequence in the dataset set is not have more than 33% sequence identity.
Table_sup2:-The performance of amino acid composition based module developed using the dataset of Karchin et al., 2002.
Table_sup3:-The number of sequences in each subfamily.
Table_sup4:-List of sequence with suggested subfamilies. These sequences are kept unclassified in GPCRDB beyond inclusion in CLASS A.
Figure_S1:-The diagrammatic view of independent or blind dataset.The numbers shown in bracket specify the number of sequences for different families and subfamilies.
Other GPCR Related Resources:-
|